Each data point is scored as positive (1), neutral (0) or negative (-1). A score is then calculated by subtracting total negative conversation from the total positive conversation (Net Sentiment = positive conversation – negative conversation). To calculate percentage Net Sentiment, this score is divided by the total number of posts (positive, neutral and negative) during that period.
Net Sentiment
A powerful real-time customer satisfaction metric
Net Sentiment is an aggregated customer satisfaction metric that is a critical component in the quest for an authentic and complete voice-of-customer. Net Sentiment is a real-time alternative to lagging metrics like Net Promoter Score and is measured by collecting unstructured text from publicly available online conversations.
The benefits of Net Sentiment
Unsolicited naturally occurring consumer feedback
Social media is an ideal and rich source of publicly available data from which to gauge public opinion. Unlike typical survey methods, the views are volunteered, without prompting on what to say or how to say it.
Real-time and leading indicator of opinion
Net Sentiment provides a continuous measure of public opinion. Traditional research methods and tools, such as NPS and focus groups, provide useful review data but are backwards-looking indicators that take time to collect and date quickly.
Benchmark against competitors and past performance
Publicly available data allows Net Sentiment to be benchmarked against your competitors and your own past performance. This enables quick course correction and informed industry and market perspective.
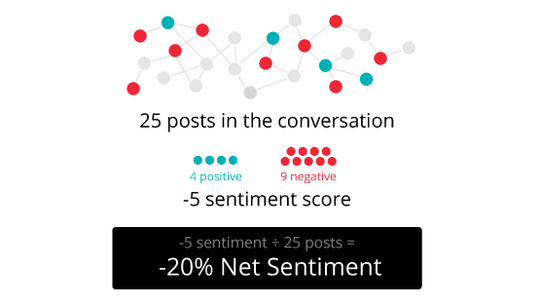
How is Net Sentiment calculated?
Differentiate operational and reputational feedback
Harnessing the DataEQ Crowd’s ability to segment data using human insight and inference, we provide a differentiated view which splits out operational and the reputational conversation.
A key challenge when understanding the root causes of Net Sentiment is to differentiate ‘reputational’ conversation – online press coverage, owned and earned PR and marketing efforts, and publicity generated by social responsibility efforts – from feedback about the customer’s experience of business operations. Customer experience conversation is often a predominantly negative space, which can mask the successes of attempts to build a brand that is viewed positively in the market.
Similarly, brand-building can only go so far in the absence of good customer experience, and understanding drivers of dissatisfaction are critical, as this conversation can undermine overall perception. Using the Crowd’s ability to segment data using human insight and inference, we provide a differentiated view which splits out customer experience conversation (operational) from the reputational conversation.
We’ve relentlessly challenged the norms of banking to create a simplified product that is built upon client-centricity. DataEQ’s data helps us keep our finger on the pulse of our clients and South Africans in general. We’re able to listen to client feedback, gain insights into unmet needs and spot trends in real-time, which helps us deliver the world-class client service and industry-leading products our clients have come to expect.
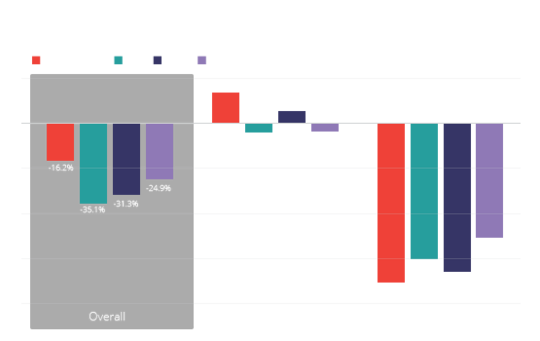
Case study
Separating operational and reputation feedback reveals a discord in the perception of brand and CX for UK telecoms
By splitting out the operational and reputational feedback of leading UK telecoms, we were able to identify the roots of positive and negative performance. The sentiment chart for February to April 2019, revealed that although Vodafone had the worst CX performance, their branding efforts drove their leading overall Net Sentiment score. This division ensures that a reading of CX performance is not skewed by marketing efforts, and vice versa.
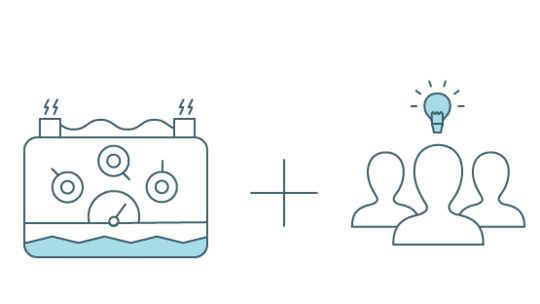
Human-verified data guarantees accurate sentiment
For Net Sentiment to be a valuable metric, it must be highly accurate and represent real shifts in customer satisfaction. Sentiment analysis has typically relied on Natural Language Processing (NLP), which has difficulty parsing the informal writing style used on social media and making inferences from context. Slang, sarcasm, emoticons and multilingual posts are challenging for NLP to contextualise and therefore the sentiment of the author may be categorised inaccurately. To overcome these limitations, DataEQ combines AI and human intelligence. Algorithms are used for certain preprocessing tasks and our Crowd of trained human contributors are able to evaluate the sentiment, contained in each social media mention.